FedS3A
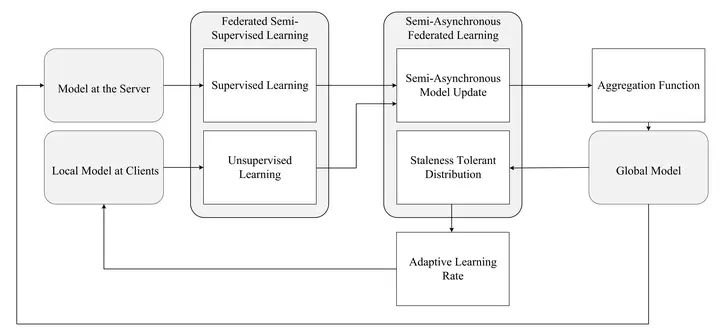
Working on the design of Federated Semi-Supervised and Semi-Asynchronous Learning (FedS3A) for anomaly detection in IoT networks, addressing a more realistic semi-supervised scenario. The framework introduces a semi-asynchronous model update and staleness-tolerant distribution scheme to balance round efficiency and detection performance. It accounts for the staleness of local models and the participation frequency of clients to ensure fair contributions to the global model. Additionally, a group-based aggregation function is employed to handle non-IID data, while difference transmission using sparse matrices is adopted to reduce communication overhead. (2022–present, submitted to the IEEE Internet of Things Journal (IOTJ) [CCF C, SCI-Q1]).